Sports Analytics Model Adapted to Improve Cancer Outcome Prediction
Erik Nelson and Anne-Marie Silvi
August 5, 2019
Outcome prediction is central to the care of cancer patients. Will the patient be symptom-free at some point in the near future or succumb to the disease? Will the patient benefit from a more aggressive or less aggressive therapy? Scientists and clinicians have spent considerable effort to define parameters to predict patient outcomes and the best treatment approaches. These efforts have improved patient care, but major challenges remain. For instance, it is common for patients with seemingly similar characteristics to have drastically different outcomes.
Limitations of Current Outcome Prediction
Most risk stratification is traditionally done prior to treatment, yet response to therapy is often a strong prognostic factor. Therefore, incorporating therapy response would likely provide a benefit to cancer patients. One marker used after treatment is minimal residual disease (MRD). MRD is a measurement of cancer cells present after treatment in patients that otherwise do not show signs of disease. MRD is an important measurement, as it can indicate who is likely to relapse. Another more recent development is the measurement of circulating tumor DNA (ctDNA) in the blood of cancer patients. During the normal course of disease, some cancer cells die and release their DNA into the blood. These small DNA fragments can be isolated and identified as cancerous according to their mutational profile, and the level of detectable ctDNA correlates with survival in at least some cancers. Ideally, ctDNA is collected before and after treatment. Though current predictive models are beneficial, risk categorization does not always correlate with outcomes, nor does it always inform clinicians which patients might benefit from more intensive therapy.
From Predicting Super Bowl Outcomes to Predicting Cancer Outcomes
To address the challenges of current risk prediction, Ash Alizadeh and Maximilian Diehn from Stanford Medical School, along with first authors David M. Kurtz, Mohammad S. Esfahani, and Florian Scherer, developed a process to integrate pretreatment and post-treatment characteristics. Importantly, this process is dynamic, since information obtained at any time can be incorporated into the model, potentially increasing the value of the assessment. This new method is presented in the latest issue of Cell.
Drs. Alizadeh's and Diehn's model for dynamic risk prediction in cancer patients is called the Continuous Individualized Risk Index (CIRI) and is modeled after predictive models used in other fields, such as sports and politics. Their team first tested CIRI in diffuse large B-cell lymphoma (DLBCL) using inputs from six parameters: the international prognostic index (IPI), cell of origin of the cancer, imaging, pretreatment ctDNA levels, and post-treatment ctDNA levels measured by early molecular response (EMR) and major molecular response (MMR). Prospectively, the IPI, cell of origin, and the pretreatment ctDNA levels would be determined when the patient is diagnosed, while the EMR, MMR, and imaging would be collected at various times during the disease course. The risk estimate would be refined and updated as new information were added.
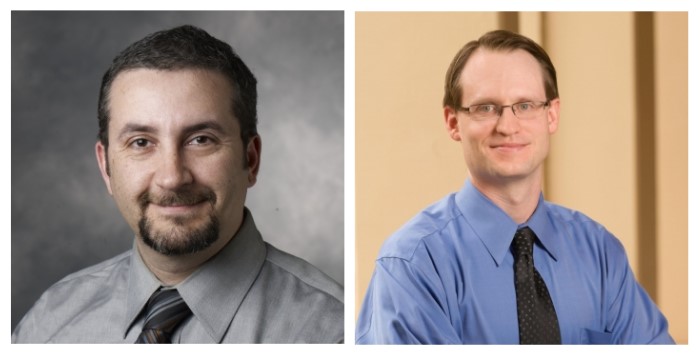
Left: Corresponding Author Ash Alizadeh, MD, PhD
Right: Co-Corresponding Author Maximilian Diehn, MD, PhD
Putting CIRI to the Test
CIRI was tested in diffuse large B cell lymphoma (CIRI-DLBCL) in a cohort of patients with all six of the risk criteria available. CIRI-DLBCL was queried to determine whether CIRI can predict event-free survival at 24 months (EFS24 – a clinically relevant endpoint in disease management). Importantly, the CIRI-DLBCL estimation very closely matched the observed likelihoods, demonstrating the accuracy of the CIRI risk prediction. Statistical analysis demonstrates that CIRI-DLBCL is significantly more accurate to predict EFS24 than current methods that use IPI, imaging, EMR, or MMR individually. CIRI-DLBCL is also superior to existing methods in predicting overall survival at 24 months (OS24).
Since patients (and physicians) are often most interested in prediction of survival at any time during the course of therapy, CIRI-DLBCL was adapted to assess for a broader range of time. The resulting prediction corresponds strongly to the observed outcomes for both event-free survival (EFS) and overall survival (OS) at any point in time from 12 to 36 months and outperformed each of the individual predictors. This prediction also provided survival graphs showing high-, medium-, and low-risk groupings. Therefore, CIRI-DLBCL is effective to predict risk at any point in time at least up to 36 months. These data show a high correlation of prediction of outcomes with actual outcomes for DLBCL.
Adapting CIRI for Other Blood Cancers
To test whether CIRI might be useful in a blood cancer that is distinct from DLBCL, it was tested in chronic lymphocytic leukemia (CLL), which has different risk factors from DLBCL. For CLL, the CIRI predictors included IPI and choice of therapy (both obtained at diagnosis) as well as multiple MRD measurements, which are obtained after therapy has started. CIRI-CLL demonstrated a high correlation of predicted survival with observed survival. It also provided a risk categorization ranging from high to low risk. CIRI-CLL showed superior prediction of EFS and OS over a range of time points compared to any individual predictor. Therefore, in two distinct blood cancer types, CIRI accurately predicts survival better than any one predictor.
Beyond Blood Cancer: CIRI in Solid Tumors
Having established the accuracy of CIRI as a predictor of outcomes in blood cancer, Drs. Alizadeh’s and Diehn's group set out to determine whether it could also be used for solid tumors. To test this, they constructed a CIRI risk model for breast carcinoma (BRCA) patients who received chemotherapy followed by surgical resection of remaining tumor tissue. The pretreatment risk factors included clinical stage of the tumor, tumor grade, estrogen receptor status, and HER2 status. After treatment, residual tumor burden was measured and integrated as a risk factor. Similarly to blood cancer, CIRI-BRCA showed an acceptable concordance between the predicted recurrence-free survival and the observed survival over 12 to 60 months. Furthermore, the CIRI-BRCA prediction is superior to the predictive ability of any factor alone, and patients can be stratified from high to low risk based on this approach. Therefore, CIRI is effective in multiple cancer types.
CIRI as a Biomarker Prediction
Thus far, Drs. Alizadeh’s and Diehn's group demonstrated the prognostic ability of CIRI. But what about CIRI as a predictive biomarker that identifies differential effects dependent on the treatment? Typically, biomarkers are gene expression, protein levels, or other molecular characteristics that have a correlation with therapeutic response. However, a quantitative risk assessment may also be used to identify biomarkers that are not dependent on molecular characteristics. The team looked at the FCR chemotherapy regimen (a type of chemotherapy using Fludarabine, Cyclophosphamide, and Rituximab) that was used in many of the CLL patients in the available datasets. When looking at MRD as an individual biomarker, both MRD-negative and MRD-positive patients are predicted to benefit from FCR over other approaches, although the predicted outcomes of MRD-negative patients are superior to those of MRD-positive patients. When applying CIRI-CLL, patients with a probability of progression >20% are predicted to benefit from FCR whereas those with a probability of progression of <20% are predicted to see no benefit. An analysis of the actual patient data bears out this prediction, thereby demonstrating that CIRI-CLL is a predictive biomarker in this context. A number of patients analyzed had negative MRD (a low-risk factor) and were therefore previously considered low risk, but the segment of these patients who also had very high IPI (a high-risk factor) were re-classified under CIRI-CLL as high risk. This created a more accurate assessment of response to FCR.
To see if CIRI would also act as a biomarker in breast cancer, the group analyzed HER2-positive breast carcinomas treated with the HER2-targeted therapies trastuzumab and pertuzumab. The team identified a group of high-risk patients who preferentially benefit from this dual-targeted therapy. Therefore, CIRI acts as a biomarker in both CLL and breast cancer, suggesting that in addition to being an effective prognostic tool, CIRI may also be an effective biomarker in multiple cancers.
Next Steps: Future Clinical Application of CIRI
The research conducted by Drs. Alizadeh and Diehn and their team demonstrates the power of using multiple variables before and after treatment to dynamically profile the probability of survival and the risk of progression in cancer patients. Importantly, they show this in diverse cancers, including two blood cancers and one solid tumor cancer. The profiling uses risk parameters specific to each of the cancers analyzed. This demonstrates that this approach is useful for any cancer in which pretreatment and post-treatment risk factors are known. The clinical experience of each patient may not provide all of the risk factors required, but CIRI is useful even in cases with some missing data. This research was done using available databases with known clinical outcomes, and it will be important to prospectively test this model in future clinical trials. An adoption of CIRI would mean that initial assessment of a patient would occur at diagnosis, but additional risk assessment would occur at various points of time during treatment. Since each patient has a unique cancer with individualized outcomes, CIRI would be a potent component of personalized medicine to help select the most effective treatment strategies for each patient.
Dr. Alizadeh is supported by The Leukemia & Lymphoma Society through a Career Development Program Scholar Award.
References
Kurtz DM, Esfahani MS, Scherer F, et al. Dynamic Risk Profiling Using Serial Tumor Biomarkers for Personalized Outcome Prediction. Cell. 2019; 178(3):699-713.
Kurtz DM, Scherer F, Jin MC, et al. Circulating Tumor DNA Measurements As Early Outcome Predictors in Diffuse Large B-Cell Lymphoma. J Clin Oncol. 2018; 36(28):2845-2853.
Click here to explore other articles on the LLS Research Spotlight.